Client
Rostelecom
Mission
Create information arrays of Earth remote sensing data as part of the work to establish boundaries of agricultural land, agricultural lands of the Volga Federal District, the Orenburg region, the Chuvash Republic, and the Republic of Bashkortostan.
Solution
The project's official description may seem technical, but the core objective was to determine land plot boundaries and the crops they support. We achieved this by collecting and processing a wealth of historical data, capitalizing on the latest advancements in technical tools, and leveraging the power of machine learning to automate data research.
As you know, research in this field has been happening for a good while now. Over the years, we've gathered a treasure trove of historical data. But what's really exciting is that, with the latest and greatest in technical tools, the industry is finally embracing the incredible power of machine learning to automate data research.
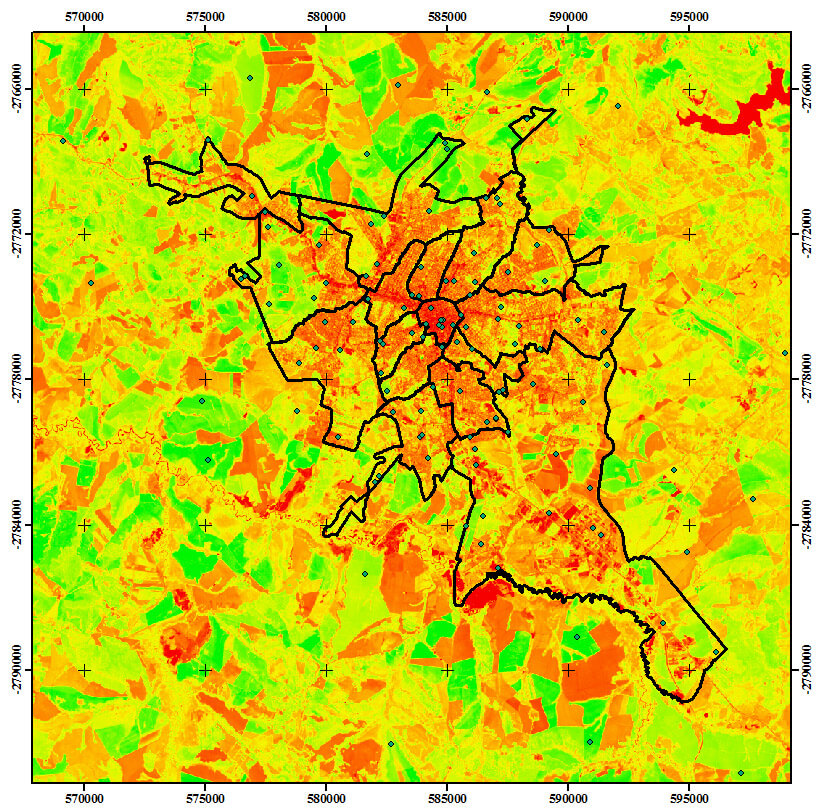
Any machine learning task always begins with data. In the scope of this project, we delved into the data available to us. For instance, apart from contemporary images, we digitized and vectorized archival data dating back to 1984. The result was a substantial dataset, comprising archival records of land plots, land survey documentation, and various types of spatial data, ranging from cartographic and geodetic maps to aerial imagery with NDVI and numerous other layers.
Through comprehensive data processing, we've constructed a historical record of agricultural land utilization. With a substantial dataset at our disposal, we've successfully developed and trained our custom neural network. This network adeptly identifies the boundaries of arable lands and classifies the types of agricultural crops they support. Following a meticulous analysis of satellite imagery, we've compiled a registry of lands recommended for inclusion in agricultural land use.
An essential consideration was the accuracy of our research. To evaluate the precision of the trained neural network model, we used a straightforward yet effective method – the creation of a confusion matrix. This matrix provided a clear assessment of the model's accuracy. In accordance with the client's specifications, we aimed for an error rate not exceeding 5%.
The project's impact extended far beyond the research itself. Updating information about agricultural lands has far-reaching significance for the entire agricultural sector. The data obtained through our research is seamlessly integrated into the Unified Federal Information System for Agricultural Land (UFIS AL). This system is an indispensable resource for government authorities and regional tax agencies. Furthermore, up-to-date information greatly contributes to the overall growth and development of the agricultural sector.
Отзыв клиента

В июле 2019 года компания ООО «Аэро-Трейд» искала подрядчика для выполнения работ по внедрению системы безналичной оплаты товаров самолетах авиакомпании «AZUR air». После тщательного изучения рынка, мы решили обратиться для реализации данного проекта в компанию ООО «МСТ Компани». Основной задачей было обеспечить каждый борт авиакомпании «AZUR air» терминалом, который сможет принимать платежи не только на земле, но и во время полёта.
В течении всего времени нашего сотрудничества, специалисты ООО «МСТ Компани» продемонстрировали отличные профессиональные навыки при подготовке проекта, и разработке документации. В результате мы получили гибкое и надёжное решение, которое удовлетворяет нашим требованиям.
По итогам работы с компанией ООО «МСТ Компани» хочется отметить соблюдение принципов делового партнерства, а также четкое соблюдение сроков работ и выполнение взятых на себя обязательств. ООО «Аэро-Трейд» выражает благодарность специалистам компании за проделанную работу в рамках внедрения системы безналичной оплаты на самолетах авиакомпании «AZUR air». И рекомендует компанию ООО «МСТ Компани» как надёжного партнёра в области платёжных решений.